We live in a world where people and businesses are more interconnected than ever before. Companies of all sizes are looking for ways to expand into new regions worldwide. However, these organizations face problems with language barriers that cause a lack of communication. Language issues can lead to significant challenges in reaching different target audiences. This is why companies have started to rely on machine translation as a tool to bridge the language gap.
This is where Machine Translation Quality Estimation (MTQE) becomes a crucial solution. It allows companies to gauge and improve the quality of their translated content quickly and efficiently.
In this article, we review how MTQE empowers businesses to manage their multilingual communications successfully with precision and confidence.
Table of Contents
What is MT Quality Estimation (MTQE)?
Machine translation has come a long way in recent years; however, MT translations may still contain errors. These errors need to be evaluated and corrected by human translators, which means additional time and effort are needed for each project. However, there is a solution, and it’s called MTQE.
Machine Translation Quality Estimation is the process used to ensure machine-translated text is the same quality as human translators. Using MTQE ensures the translation process is faster because it only focuses on text that contains potential errors. This evaluation method uses machine learning to predict which text segments are accurate or in error. A human translator corrects those that are not accurate.
MTQE requires the development of specific policies, metrics, and the use of tools to measure the quality of machine translation-generated texts.
MT Quality Assessment Tools
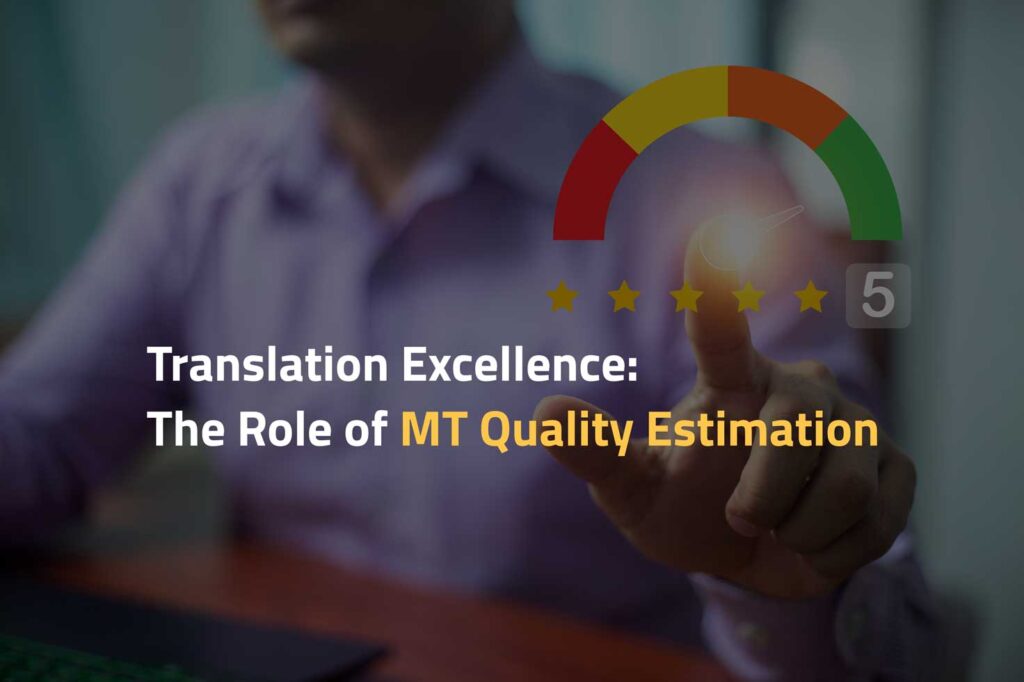
MTQE uses several tools and metrics that improve the quality of machine-translated content, including:
Automatic Translation Evaluation (ATE): this process uses algorithms to create quality scores, such as BLEU (Bilingual Evaluation Understudy), TER (Translation Error Rate) in MT, and METEOR (Metric for Evaluation of Translation with Explicit Ordering) based on reference translations.
Quality Scores for Machine Translation: are numerical ratings assigned to MT-translated text that evaluate its accuracy, fluency, and quality. The scores are determined by different automated evaluation metrics and algorithms designed to assess the quality of MT translations.
MT Confidence Scoring: estimates the quality level of machine translation systems for the correctness of their translations. It also helps users in assessing the reliability of the translated text.
Predictive Quality Models: use predictive analytics and machine learning to determine translation quality based on linguistic features, context, and historical data.
Human vs. Machine Translation Quality Comparisons: side-by-side evaluations are done to compare human-translated content and machine translation of the same text. This comparison identifies areas where machine translations need to be improved.
MT quality metrics are used to measure the accuracy, fluency, and quality of the MT-translated content. These metrics are essential for:
1. Checking the texts to ensure their quality is good for real-world use.
2. Acting as a guide for research and development.
Machine translation is not static; the technology continually learns and improves over time. These metrics are used to ensure the systems are working as expected, though they can also help to enhance the translations produced by the machine systems.
The Role of AI and Machine Learning
AI (Artificial Intelligence) and Machine Learning (ML) are essential to modern translation work. These technologies use automated evaluation methods, enabling more precise and efficient translation quality assessment.
AI and machine learning will continue to play a significant role in MTQE by creating more accurate evaluations. These evaluations can be used to improve MT technology. Together, AI and machine learning will also improve multilingual communications between business partners and customers.
The Importance of Human Evaluation
Human evaluation remains essential in Machine Translation Quality Estimation for several reasons. For one thing, humans use their subjective judgment and have more linguistic experience that comes to bear translation quality. They can detect cultural nuances, references, and idiomatic expressions that machine translations may overlook. In addition, human linguists also have a better understanding of translation quality and consider other factors that go beyond literal translations.
Moreover, human evaluation sets the standards for determining whether the performance of automated evaluation metrics is correct. Comparing human-translated text and machine translations allows MTQE to be validated and updated. This ensures that the systems provide quality translations on par with human translations.
Humans are also better at domain expertise, determining error analysis, and quality assurance. While machine translations are now of higher quality than in the past, human linguists are still necessary to ensure the quality of machine-translated texts. In this way, errors and mistranslations are reduced. However, working together, human and machine translation methods provide more accurate, reliable, and user-friendly translation services.
Conclusion
Machine Translation Quality Estimation will continue to play an essential role in the challenges and opportunities faced by the growing demand for multilingual content. With automated evaluation metrics, human translator assessments, and industry standards, MTQE will continue to offer accurate, reliable translations in multiple languages and domains.
As machine translation technologies continue to advance, translation quality will continue to improve in the future. However, to ensure machine translations are high-quality, combining machine translation and human translation services is recommended for the best outcome.
Read more
- Audio and Video Translation
- E-learning Translation: Meeting the Demands of the Global Market
- Translators and Interpreters: Bridging the Language Gap
- Effective Translations and Localization for Middle Eastern Countries
- Navigating Business and Language Diversity in Iraq
- Celebrating World Arabic Language Day
- Maximizing Language Services for Success: Essential Strategies
- Should You Translate Your Brand Name?
- Can You Learn English with AI?
- Common Localization Mistakes to Avoid for a Hassle-Free Global Launch
- Beginning with Email Translation and Customization: A Guide for Beginners
- Debunking Common Translation Myths
- The Power of Translation Memory: A Guide
- Global Success for an E-Commerce Marketing
- Bridging Barriers to the Kurdish Language
- Introducing the Languages of the World
Machine Translation FAQs
How is machine translation quality measured?
Machine translation quality is measured through both automated evaluation methods like BLEU, METEOR, and TER, and human evaluation, focusing on readability, fluency, and accuracy compared to the original meaning.
What tools are available for assessing machine translation accuracy?
Tools like BLEU, METEOR, TER, NIST, and WER are used to assess machine translation accuracy, supplemented by human translators for final quality checks.
Can machine translation quality match human translation?
While improving, machine translation (MT) does not yet match the quality of human translators, particularly in handling complex expressions and maintaining accuracy.
What factors affect the quality of machine translations?
Factors affecting MT quality include the choice of MT software, the domain or industry specificity of the content, content type, and the language pair involved.
How can machine translation quality be improved?
Improving MT quality can be achieved by using simple, clear language, training the MT system with domain-specific data, and refining the processes over time.
Are there industry standards for machine translation quality assessment?
Yes, standards like ISO 9000, EN 15038, and ASTM F2575-14 guide quality processes, resources, and requirements for quality translations.
These translation industry standards and frameworks provide invaluable guidance and resources for organizations and their customers in machine translation projects. These standards also ensure consistency, transparency, and translation quality. They go a long way in improving the use of machine translation technologies.